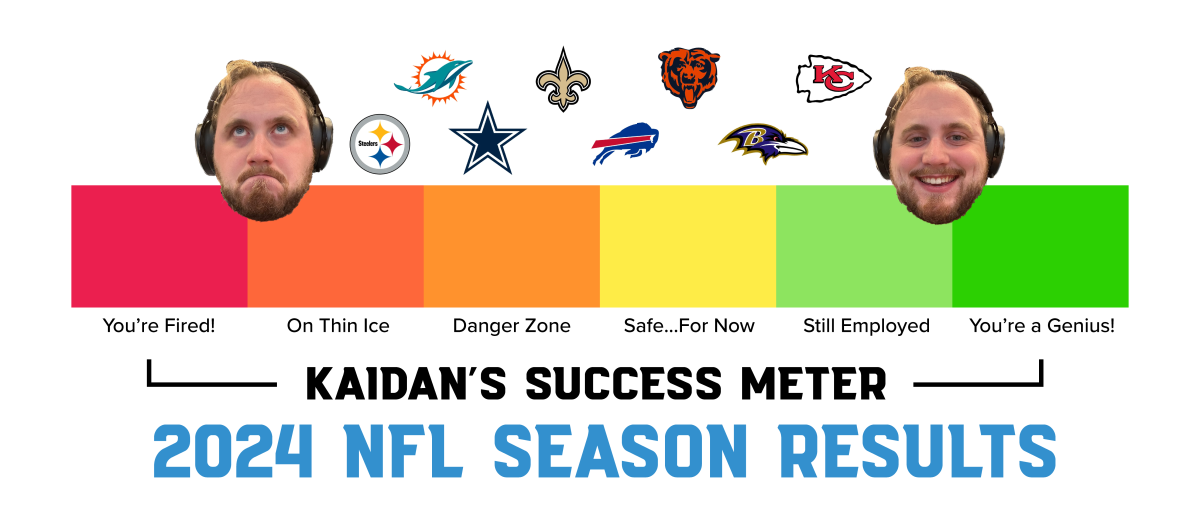
A Fun Dive into Machine Learning: Wrapping Up the 2024 NFL Season
A Look Back at the Performance of My NFL Game Prediction Machine Learning Model
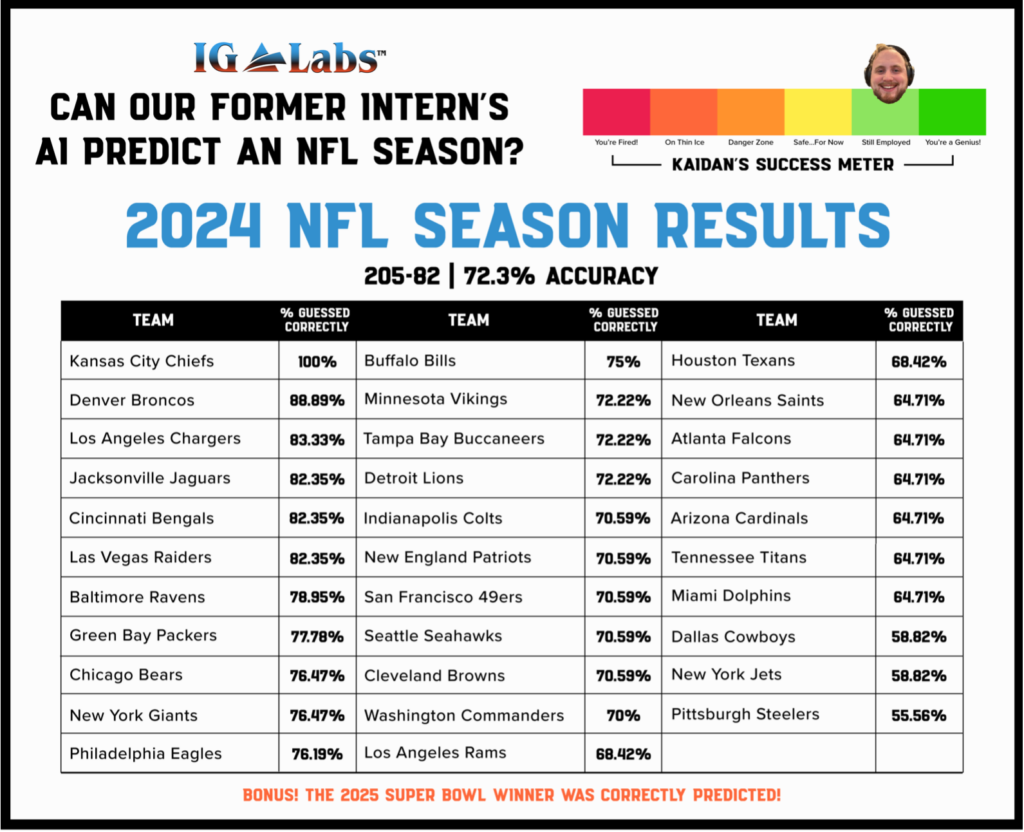
The Background
A few years ago, I started an internship project at IG Labs combining my love of football with data science, and it soon became a real challenge—could my machine learning model stand up to public scrutiny for the 2024 NFL season?
I designed the model to predict NFL game winners using open-source data on team statistics and betting odds from several gambling services. Over the years, I’ve maintained and tweaked the model, which delivered a 65% accuracy rate going into the 2024 season. The details on my model can be found here.
As a fun experiment, IG Labs published my model’s weekly predictions on our social media. Now, with the season wrapped up, it’s time to review the model’s performance, highlight some interesting takeaways, and evaluate some changes to the model for next season.
The Results
While some weeks were rough—Week 3’s dreadful prediction accuracy of 37.5% comes immediately to mind—my model’s overall performance for this season was significantly better than years past.
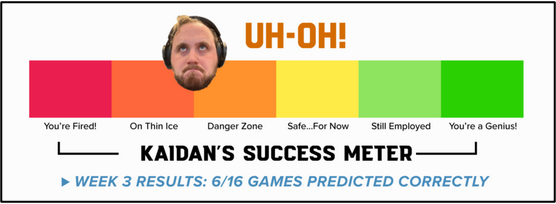
Painful memories…but my model redeemed itself!
Overall, the model’s record for the 2024 season was 205-82 on predictions—an accuracy rate of 71.4% (72.3% including the Super Bowl)!
The Highlights
Team Accuracy
One of the standout achievements of the model was to perfectly predict the Kansas City Chiefs’ season—including their Super Bowl defeat. Its performance for the Denver Broncos was similarly strong at 88.2%, only missing two of the teams ten wins, while correctly forecasting all seven of their losses.
The model also correctly predicted all of the wins for the Los Angeles Chargers, Cincinatti Bengals, Baltimore Ravens, and San Francisco 49ers, as well as all of the Denver Broncos’ losses.
Conversely, the model struggled with the Pittsburgh Steelers, with a 55.6% accuracy rate in predicting the team’s wins and losses—only picking four of the team’s ten wins. Its performance with the New York Jets and Dallas Cowboys wasn’t much better: 58.8%.
It also failed to pick any of the wins—albeit few—for the New York Giants, New England Patriots, and Tennessee Titans. Likewise, it missed all of the losses for the Philadelphia Eagles and the Detroit Lions.
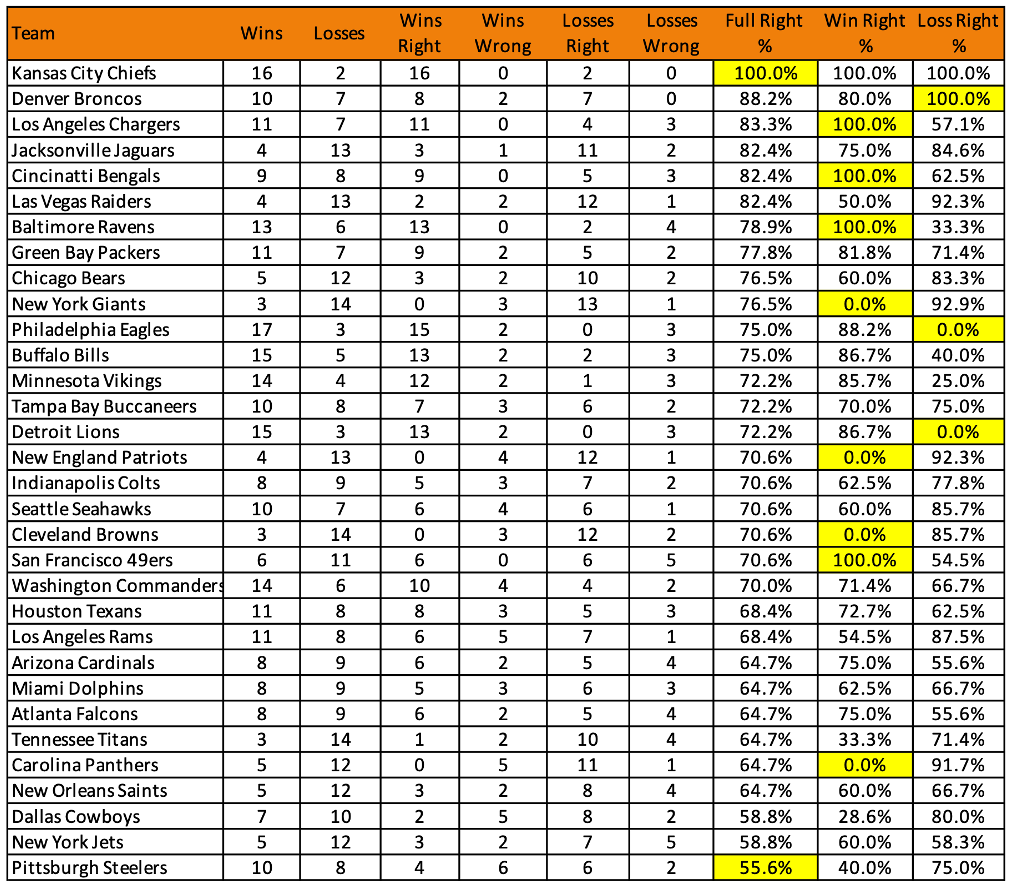
Note: data includes post season games and the Super Bowl
Post Season Predictions
The model also made playoff seed predictions each week. The AFC seed picks were strong, especially the Kansas City Chiefs as the #1 seed and the Houston Texans at #4. As well, the Buffalo Bills and Baltimore Ravens were almost equally represented as the #2 and #3 seeds. The NFC landscape was a bit trickier, though, with the exception of the Philadelphia Eagles, who were the #2 seed for 10 weeks.
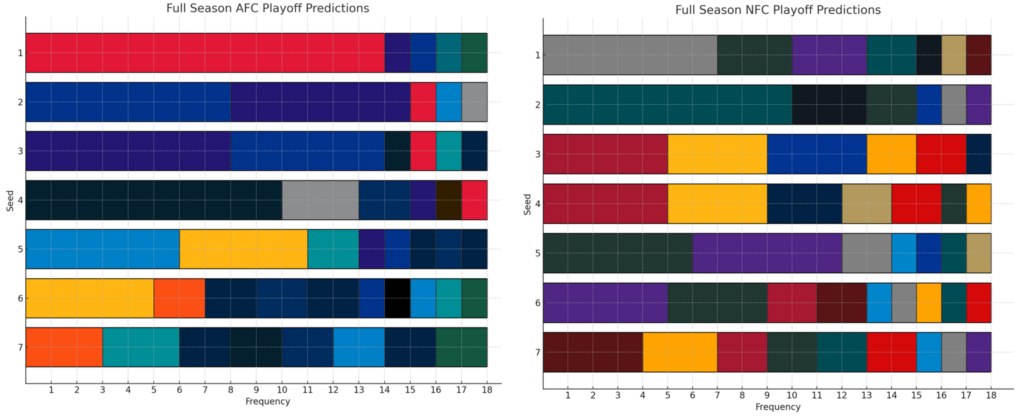

The model also made weekly predictions for the Conference Champions and the Super Bowl winner.
The model heavily favored the Chiefs, Ravens, and Bills for the AFC Champion, picking each team seven, six, and five times respectively. For the NFC Champion, the model was more dispersed in its picks, with the Eagles being the favorite for six weeks of the season, followed by the Lions for 4 weeks
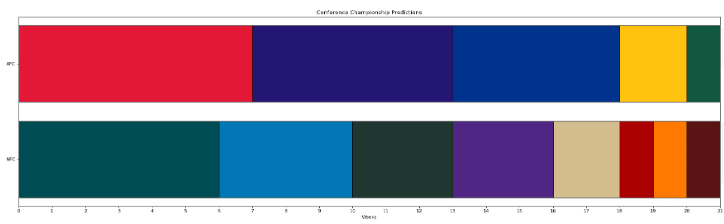
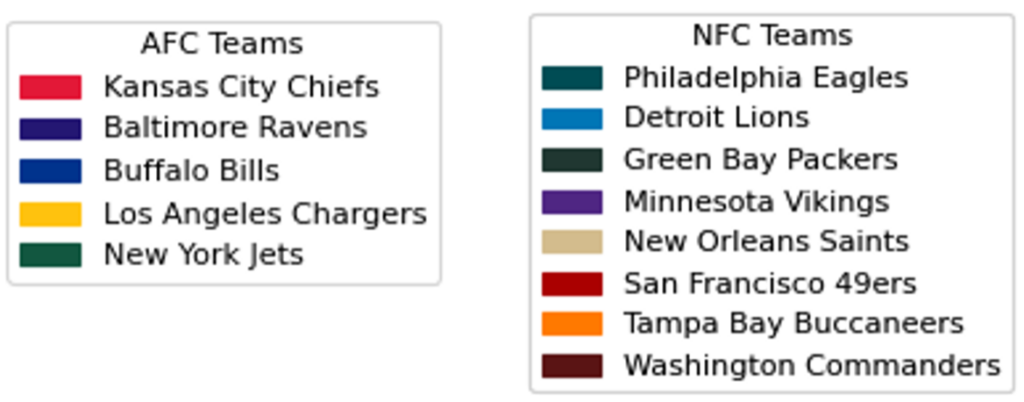
Super Bowl Predictions
The model’s Super Bowl matchups varied a lot from week to week, but its projected winners were fairly consistent. The actual winners—the Philadelphia Eagles—were picked as winners four times, while the losing Kansas City Chiefs were picked six times.
The Buffalo Bills and Baltimore Ravens were each projected to win the Super Bowl four times during the season.
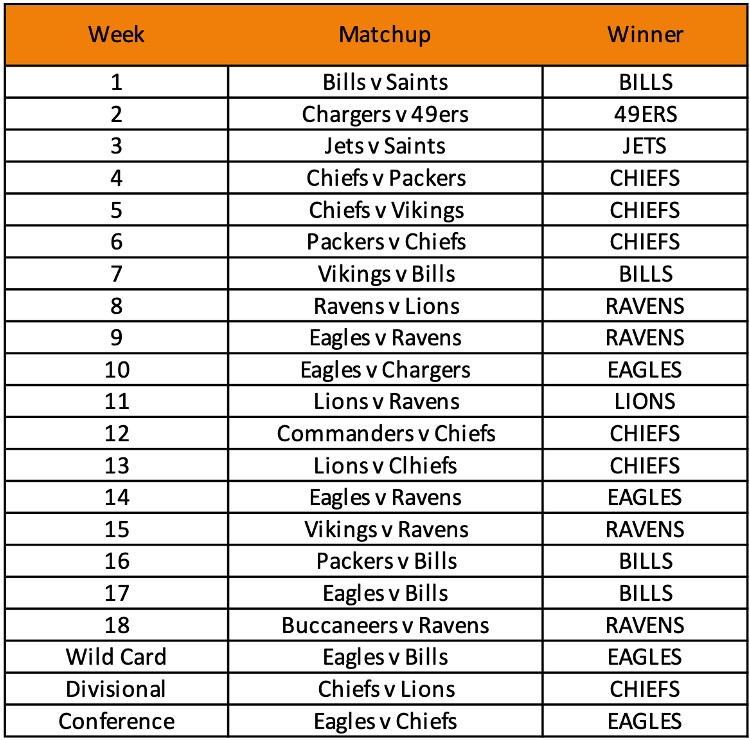
Changes for Next Season
This was the first season I incorporated betting data, and it was a big success! It improved accuracy by about 6% and, even though this is an accomplishment, I think the way betting data is utilized can be better.
To start, the week’s games were predicted every Thursday (since the earliest game every week is Thursday). If the betting data were to change drastically between Thursday and Sunday, it would not be reflected in the model. Discovering a consistent time to add in all the betting data in relation to the game could improve the model’s accuracy.
A more significant change I’ll be implementing is to only apply the data to one of the models. The NFL Model is three separate models working independently, all using the same data. One model predicts total points, one model predicts a winner, and one model predicts a point differential. If the projected winning team does not align with the team that is projected to get more points, the more extreme guess is used.
As the season progressed, my model’s predictions became less distinguishable from the betting odds. That being, the team that was the betting favorite to win would be predicted to win almost every time. There are only three instances since week 10 that the model correctly predicted the outcome of a game that went against the betting odds—89% of all incorrect predictions occurred on games where the betting favorite did not win.
To fix this, next season I will only feed betting data to the model dealing with the point differential, while keeping the model that predicts a winner separate. Hopefully, this will shake things up a little bit and give my model a better chance of guessing the upsets.
Overall, this year’s models performed reasonably well—72.3% accurate—so I get to keep my job! Working with the models throughout the season also provided me with great insights into how they operate and how the input data can influence the resulting predictions. Even though the games and excitement are over for now, I’ll continue to think of other modifications during the off-season to improve next year’s performance.