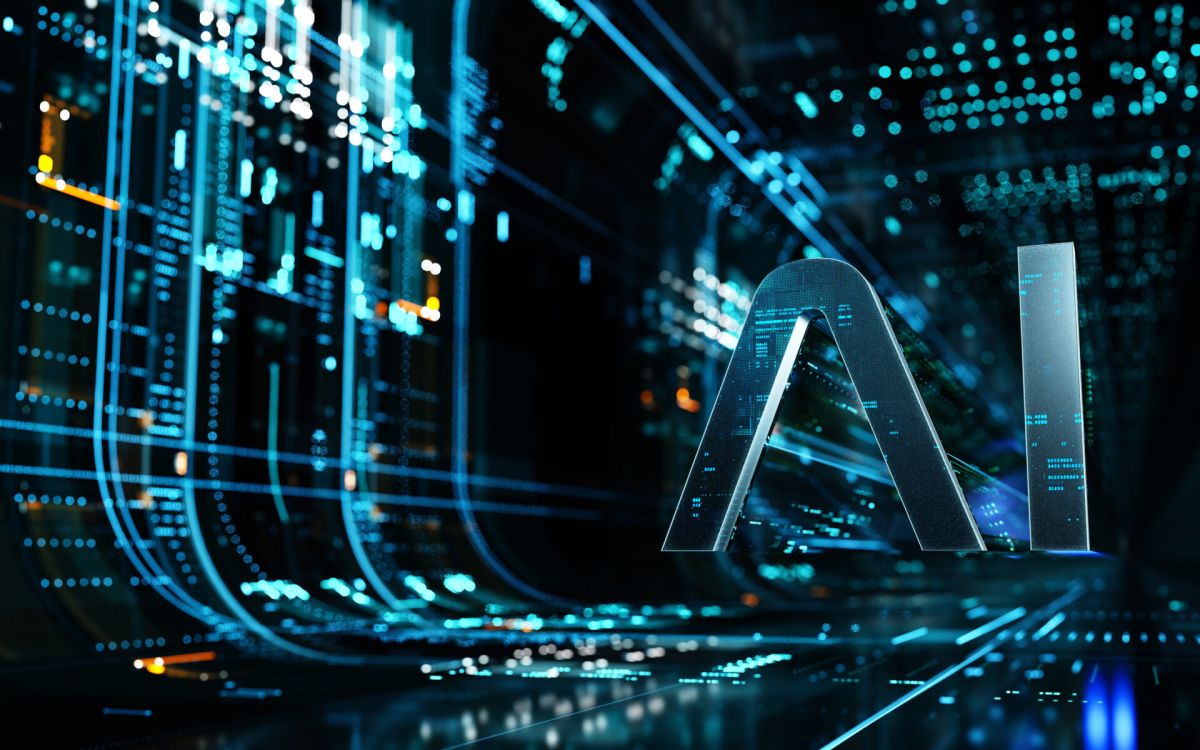
How AI and Machine Learning Power Modern NDR Solutions
The Evolution of Network Security
Cybersecurity is an evolving game, where defenders must anticipate and counteract threats before they escalate. As networks expand across on-premises, cloud, and Internet of Things (IoT) environments, the challenge of detecting and mitigating threats has never been greater.
Traditional security tools like firewalls and signature-based Intrusion Detection Systems (IDS) rely on static rules and known attack signatures—great for documented threats but ineffective against novel attack tactics and zero-day exploits.
This is where Artificial Intelligence (AI) and Machine Learning (ML) step in, transforming Network Detection and Response (NDR) from a reactive tool to a proactive defense mechanism. In fact, recent academic research on the use of AI and ML in cybersecurity applications found that implementing these methods outperformed traditional security tools in terms of anomaly detection.
AI and ML in NDR Solutions
Fundamentally, AI and ML enable NDR solutions to detect, prioritize, and respond to cybersecurity threats in real-time by analyzing massive volumes of network traffic data, baselining normal network behaviors, and identifying subtle anomalies that traditional security tools and human analysts might miss. In addition, AI and ML algorithms can predict potential future attacks by recognizing patterns that have led to breaches in the past.
Understanding the Difference Between AI and ML
AI refers to systems that mimic human intelligence, including reasoning, problem-solving, and decision-making. ML is a subset of AI and learns from data without explicit programming.
In cybersecurity, AI and ML work together to create smarter, more adaptive security solutions. ML provides the foundation by learning from network activity. AI then takes this information and makes higher-level decisions, correlating different data points, explaining threats in understandable terms, and even predicting future attacks.
For example, ML can flag an unusual data transfer as suspicious, and AI can analyze the context, determine whether it’s a real threat, and suggest an appropriate response.
How CYBERSPAN® Uses AI and ML
CYBERSPAN® leverages AI and ML to enhance threat detection and response, while simplifying cybersecurity management. Here’s how:
Machine Learning for Anomaly Detection
CYBERSPAN® employs both supervised and unsupervised learning to identify network anomalies:
- Supervised learning is used for threat detection and traffic analysis.
- By mapping malicious network traffic to known MITRE ATT&CK tactics, techniques, and procedures (TTPs), CYBERSPAN® provides context and actionable intelligence.
- CYBERSPAN® analyzes traffic sizes, flagging unusual data transfers that may indicate malicious activity.
- Using Natural Language Processing (NLP) and decision trees, CYBERSPAN® examines user agent strings for inconsistencies.
- Unsupervised Learning is used to detect anomalies without prior knowledge of “normal” network activity.
- Packet clustering identifies unusual traffic patterns by analyzing metadata such as IP addresses, ports, protocols, and byte sizes.
- Device clustering detects anomalous activity on a single device.
- Time clustering pinpoints specific time periods that contain unusually high levels of anomalous activity, helping security teams focus their investigations.
AI-Driven Insights and Actionable Intelligence
CYBERSPAN® leverages AI to provide actionable insights and automate key aspects of the incident response process:
- Threat Prediction: CYBERSPAN® leverages the MITRE ATT&CK framework to predict potential future threats based on historical attack vectors.
- Event Correlation and Contextualization: CYBERSPAN® correlates events from local sensors to provide enterprise-wide awareness of threats and patterns. It analyzes data from multiple sources, including network traffic, threat intelligence feeds, and vulnerability databases.
- Explainable AI (XAI): To empower less technical personnel, CYBERSPAN® provides a description of what was observed, why an alert was generated, and which models contributed evidence, then it recommends mitigation strategies.
- Automated Remediation Recommendations: CYBERSPAN® suggests mitigation steps such as patching vulnerabilities, isolating compromised systems, or blocking malicious IP addresses.
- Community-Driven Defense: CYBERSPAN® aggregates anonymized organization data to identify sector-specific attack trends, which can help companies anticipate threats targeting their industry.
The Result: Enterprise-Grade Protection for SMBs
CYBERSPAN® proves that AI and ML aren’t just for large enterprises. By merging supervised threat mapping, unsupervised anomaly detection, and AI-driven analytics, CYBERSPAN® delivers a powerful, user-friendly, and affordable NDR solution that helps Small to Medium Businesses (SMBs) protect their networks from evolving cyber threats.